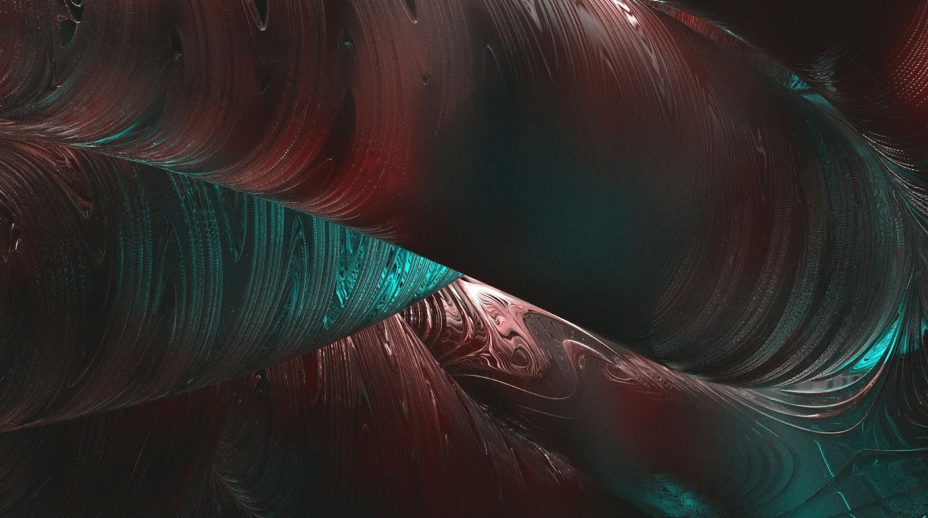
Photo by Rick Rothenberg on Unsplash
Automated data collection is changing how businesses gather and process information. It offers both speed and scalability. However, human intelligence remains essential in areas where automation falls short.
Human expertise remains vital, even with advanced algorithms. It helps manage complexity, ensures data accuracy, and makes judgment calls that machines can’t. This article explains why the human factor still plays a key role in data collection.
The Limits of Automation in Data Collection
Automated data collection is fast and efficient, but it has its limits. While algorithms handle repetitive tasks well, they often miss the context or subtle details that annotators catch easily.
When Algorithms Miss the Mark
Automated systems can struggle in areas like:
- Understanding context: Machines can’t grasp the full situation or environment.
- Spotting patterns: Algorithms may miss important trends or outliers.
- Making subjective decisions: Tasks like analyzing emotions or vague responses need an expert’s judgment.
For instance, when using data collection field services, an algorithm might misinterpret a response. An expert can correct it and improve the overall data.
Data Quality Issues: Where Human Intervention Matters
No matter how good they are, algorithms can still make mistakes. Human input is needed to:
- Validate data: Annotators can spot mistakes that machines miss.
- Ensure consistency: Annotators can catch errors that algorithms overlook.
- Fix ambiguity: Incomplete or unclear data often requires human judgment.
For better data accuracy, data collection services combine automation with human expertise to catch these issues and improve overall quality.
The Value of Expert Knowledge in Complex Data Tasks
Some data tasks are beyond the capabilities of machines alone. People have skills that algorithms can’t match. This is true for data needing deep understanding or careful judgment.
<h3>Real-World Examples
In fields like healthcare, legal, or research, human intelligence is key for gathering data.
- Healthcare: In medical data collection, interpreting patient symptoms, history, and behaviors requires a level of judgment that algorithms can’t match. Human experts ensure the data is relevant and accurate.
- Legal: When collecting legal documents or evidence, human experts understand context and can distinguish important details that automated systems may overlook.
- Research: In academic or market research, experts apply knowledge to select the right data points and adjust methodologies based on what they know, something that automated systems can’t always do.
These examples show that in areas needing accuracy, personal input in data collection is very valuable.
The Expertise Gap in Fully Automated Systems
Even though machine learning and AI have great potential, they rely on humans to guide their training and refinement. Algorithms can’t think critically or adjust to unexpected situations without human input. Experts are still needed to:
- Prepare data for training: Machines can’t decide what data is most relevant for training models.
- Monitor data quality: Human oversight is essential to spot flaws in automated systems.
- Refine algorithms: Experts play a role in improving the systems by providing feedback and making adjustments.
Without human intelligence, fully automated systems are limited in what they can achieve.
How Humans and Machines Can Work Together
Automation and human intelligence don’t need to compete—they can complement each other. The best data collection processes combine the efficiency of machines with the expertise of annotators.
The Best of Both Worlds: Hybrid Models
By using a mix of automation and human input, businesses can get the best of both worlds:
- Efficiency: Automation handles repetitive tasks quickly, allowing people to focus on complex tasks.
- Accuracy: Experts oversee data quality, correcting errors and ensuring consistency.
- Flexibility: By providing context and judgment, annotators assist machines in adapting to unfamiliar or unforeseen situations.
In big data collection projects, automated systems gather most of the data. Then, people check and confirm its accuracy. This reduces errors and increases reliability.
Building Better Systems with Expert Feedback
Machines get smarter when they’re trained and refined with expert feedback. People enhance data collection techniques and adjust algorithms to get better, more accurate results.
Experts review data and give input. This leads to ongoing improvements. As a result, systems adapt and become more effective over time.
The Future of Data Collection: Where Humans Still Fit In
As automation in data collection becomes more advanced, the role of people may seem less important. In the end, human intelligence is crucial for tackling complex tasks and ensuring high-quality data.
Predictions for the Future
In the future, machines will continue to take on more data collection tasks, but humans will still be critical for:
- Supervising automation: Ensuring algorithms are working as expected and handling exceptions.
- Handling edge cases: Addressing situations that the system isn’t designed to handle.
- Interpreting ambiguous data: Understanding data that requires deeper insight or context.
There will always be a need for human oversight in the creation and refinement of data collection methods. Automated systems need human help. Without it, they find real-world data too complex.
How to Future-Proof Data Collection
To stay ahead of the curve, businesses should focus on learning how to collect data, combining automation and human oversight. This hybrid approach lets the system collect large amounts of data. It also keeps accuracy and quality high.
By continuing to value the human factor in data collection, you’ll set your business up for long-term success.
Balancing Speed and Quality in Data Collection
While automation speeds up data collection, it can sometimes compromise quality. That’s where human expertise comes in. Humans can spot errors, correct inconsistencies, and provide deeper analysis, all while ensuring the data remains reliable.
In data collection, speed is important, but so is accuracy. A purely automated system may gather data quickly, but without oversight, it risks missing key details or making mistakes. Human involvement ensures the quality doesn’t suffer as you scale up operations.
For businesses that rely on data to guide decisions and outcomes, maintaining a balance between speed and accuracy is key.
Conclusion
Despite the growing power of automated data collection, human intelligence remains indispensable. Machines excel at handling large amounts of work and repetitive tasks. But they can’t match the nuanced judgment, expertise, and oversight that humans offer.
Success in data collection depends on merging automation with human knowledge as it continues to evolve. This hybrid approach will provide reliable, accurate, and insightful data. This sets businesses up for long-term success.